Digital Twins are Ready for Prime Time
Raymond LeClair
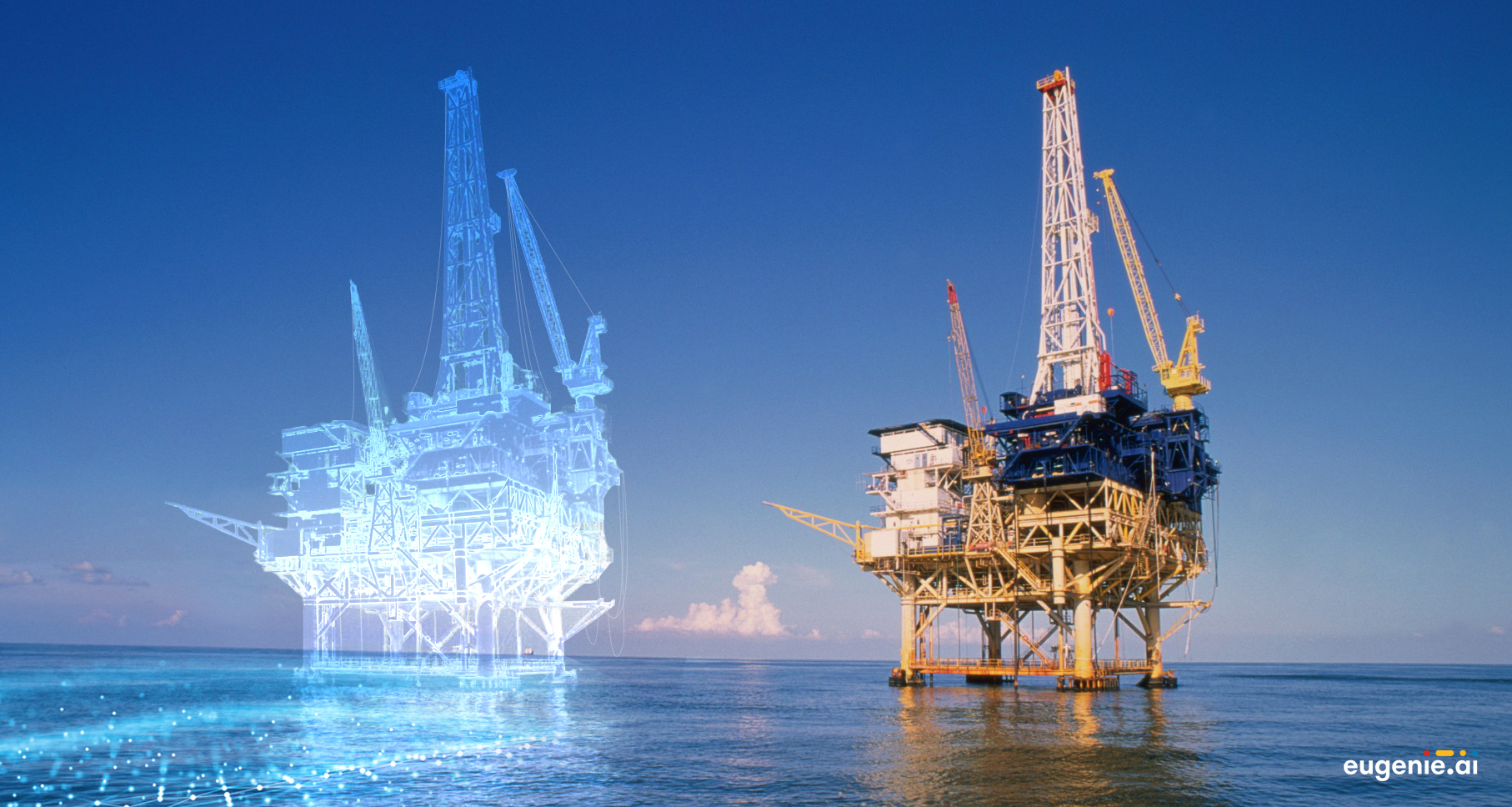
Have you ever noticed that the future is shown in blue? I didn’t until looking for images representing digital twins. And then I was flooded with blue hued images, one of which is shown above. There are interesting cultural and scientific reasons why emergent technology is often depicted in blue. More on that another time, but for now, let’s talk about digital twins, which are at least as interesting…
What is a digital twin?
Michael G. Kapteyn, Jacob V. R. Pretorius, and Karen E. Willcox provide a definition in their Nature Computational Science article “A Probabilistic Graphical Model Foundation for Enabling Predictive Digital Twins at Scale.”
“A digital twin is a set of computational models that evolve over time to persistently represent the structure, behavior and context of a unique physical asset.”
In simple terms, a digital twin is a digital representation of a specific real-world entity. Here are some real-world examples: digital twins have recently been developed in the small for the wing of an aircraft, and in the large for an offshore platform. While a topic of research and development for many years, digital twins are coming into their own, and may very well represent the future for developing and managing real world assets. Structural digital twins have shown promise in virtual health monitoring, certification, and predictive maintenance. Digital twins of human beings promise to advance medical assessment, diagnosis, and personalized treatment. Indeed, digital twins have been recently developed for continuous health monitoring, and for fitness management, though some raise ethical concerns. And smart cities enabled by digital twins and Internet of Things (IoT) devices promise to revolutionize urban planning by providing predictive insights into the city’s performance.
Why now?
A number of technological developments are converging that enable digital twins. Surely one of the most significant is the explosion of networked devices that provide data describing their internal state, and using sensors that provide data describing their environment. This is the so-called Internet of Things (IoT). According to IoT Analytics, the global number of connected IoT devices will grow to 12.3 billion by the end of 2021. And according to Market Watch, the global Internet of Things (IoT) market in manufacturing is valued at USD 165.27 billion in 2021.
Dealing with all of the data produced by these devices requires software for distributed real time processing, and availability of high performance computing. On the software front, Apache Flink, a framework and distributed processing engine for stateful computations over unbounded data streams, is a noteworthy development. Applications developed using Apache Flink have processed trillions of data ingest events per day, maintained terabytes of state, and run on thousands of cores. Or course, in order to build applications at this scale, developers need access to the appropriate hardware. But with the maturity of cloud computing resources such as Amazon Web Services (AWS), Microsoft Azure, and Google Cloud Platform (GCP), these resources are now readily available.
Obstacles to development and adoption
There are obstacles to the development and adoption of digital twins, however. Cost and complexity of development appears to be one such obstacle. Consider the European Union’s Destination Earth project which “aims to develop a high precision digital model of the Earth to model, monitor and simulate natural phenomena and related human activities.” The initiative will be implemented by the European Space Agency (ESA), the European Centre for Medium-Range Weather Forecasts (ECMWF) and the European Organisation for the Exploitation of Meteorological Satellites (EUMETSAT) over the next 7-10 years. Coordinating the development of models as diverse as required to model climate change, the state of the oceans, the cryosphere, biodiversity, land use, and natural resources among multiple organizations is a complex and costly project.
Overcoming this complexity will be a key development for the continued development and adoption of digital twins. A number of prominent software companies, among them Microsoft) and Autodesk, have begun to offer platforms for building digital twins for the Architecture, Engineering and Construction (AEC) industry, which are promising. Still, these platforms are not of general applicability, and so the mathematical formulation developed by Kapteyn, Pretorius, and Willcox is a welcome attempt to move from one-off digital twins built through custom implementations to robust digital twin implementations at scale. The authors propose a probabilistic graphical model as the formal mathematical representation of a digital twin and its associated physical asset.
New to me, probabilistic graphical models appear to be an active area of research and practice. Searching online, one can find a Stanford University specialization in probabilistic graphical models by Daphne Koller, one of the founders of Coursera, a massive open online course provider, and an MIT Press offering, Probabilistic Graphical Models, also by Daphne Koller with Nir Friedman. Wikipedia describes these models as using a graph to express the conditional dependence between random variables. In the simplest sense, think of a decision tree: a diagram in which nodes represent a condition for which a choice is required, each branch represents an intermediate outcome of the choice, and each leaf node represents a final outcome.
The authors identify two main results of their work. The first main result is the abstraction of a digital twin and its associated physical asset into a representation composed of six key quantities: digital state, control inputs, physical state, observational state, quantities of interest, and the reward. And the second main result of work is the development of a probabilistic graphical model which encodes the interaction and evolution of these quantities. The authors claim that their mathematical formulation advances the field of digital twins by defining the elements comprising an abstract representation of an asset–twin system, identifying the interactions between elements that need to be modeled, and incorporating end-to-end uncertainty quantification via a Bayesian inference framework.
Significant Investment
Whether this claim will prove true or find wide applicability or not, significant investments are being made in digital twin technology. For example, in addition to its AEC offering, Autodesk announced the $1B acquisition of Innovyze, a digital twin for water resource management, in February, 2021. And a recent study from Juniper Research projected total global spend on Digital Twins will reach $12.7 billion.
So what is the bottom line? Springbok believes this investment, fundamental research, maturing technology, and increasing industry adoption will lead to widespread use of digital twins for both scientific and commercial purposes. As our contribution to the technology, Springbok is developing a digital twin of the space domain. Of course, our digital twin will be blue.